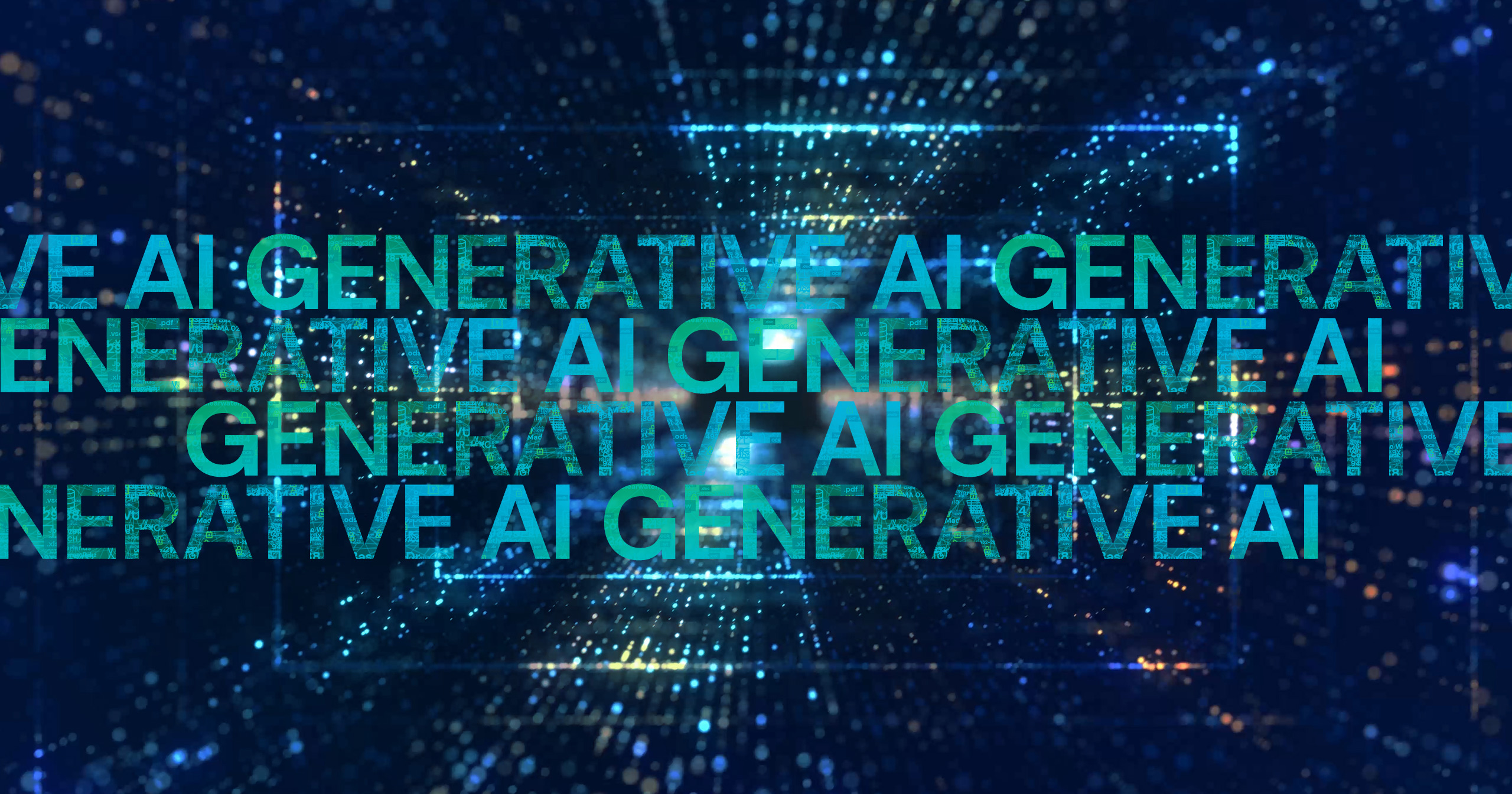
BY SPIRION
June 20, 2024
In our recent webinar, “How to Reduce Data Risk and Deliver Value with Generative AI,” we gathered a team of cybersecurity C-Suite experts to delve into the transformative potential and risks of generative AI in the realm of data security. They included Steve Hindle, CISO and Founder of Achiles Shield; Raffaele Mautone, CEO, Judy Security; Christina Shannon, CIO, KIK Consumer Products; and Rob Server, CTO, Spirion. These are the key points and insights shared during the session.
Understanding the Role of Generative AI in Data Security
Generative AI, with its advanced algorithms and machine learning capabilities, can significantly enhance data security measures. It is adept at identifying patterns and anomalies within large datasets, which is crucial for detecting potential security threats and vulnerabilities.
Key Benefits:
- Enhanced Data Discovery and Classification— Generative AI automates the process of discovering and classifying sensitive data, ensuring all critical information is accounted for and appropriately managed. This reduces the risk of data breaches by maintaining robust data governance frameworks.
- Risk Reduction— The predictive capabilities of generative AI allow organizations to anticipate and mitigate risks before they materialize. By analyzing historical data and recognizing trends, AI can provide early warnings about potential security issues.
- Improved Compliance and Regulatory Adherence— With evolving data protection regulations such as GDPR and CCPA, compliance is more critical than ever. Generative AI aids in maintaining compliance by continuously monitoring data practices and ensuring they meet regulatory standards.
- Cost Efficiency and Operational Excellence — Automating data security processes with generative AI not only reduces the manpower required but also minimizes human errors. This leads to significant cost savings and more efficient operations.
Best Practices for Data Security While Using AI
1. Accurate Data Classification:
- Ensure data is accurately classified and enriched before it is used in AI models. This step is crucial for targeting security measures effectively.
- Enrich data with appropriate and persistent tags that ensures only clean data enters AI tools.
2. Data Pseudonymization and Cleansing:
- Proactively manage data by pseudonymizing and cleansing it before it is ingested by AI systems to minimize the risk of data breaches.
3. Continuous Evaluation of AI Governance:
- Regularly review and update AI governance policies to adapt to new technologies and threats. Quarterly evaluations can help keep governance frameworks relevant and effective.
4. Combining People, Process, and Technology:
- Security should not rely solely on technology. A comprehensive approach that includes people and processes is essential for robust data protection.
5. Collaboration Across Teams:
- Establish cross-functional teams to oversee AI projects and ensure security protocols are integrated from the outset.
6. Data Minimization and Governance:
- Implement strict data governance frameworks to classify and minimize data, ensuring only essential data is retained and used in AI models.
7. Security by Design:
- Adopt a security by design approach where security protocols are embedded into AI development processes from the start.
8. Understanding Data Flow:
- Conduct thorough data discovery and classification to understand data flow and implement controls to manage and secure data throughout its lifecycle.
Audience Questions and Panelist Answers
During the Q&A session, several pertinent questions were raised by the audience, providing further insights into practical data security strategies:
Q: How can organizations start with AI initiatives without compromising on security?
- Christina Shannon advised starting small with AI projects and incorporating security by design. She emphasized not waiting for a perfect solution but iteratively improving security measures.
Q: What are the key considerations for data governance in AI?
- Steve ‘Stitch’ Hindle highlighted the importance of accurate data discovery and classification, ensuring data governance policies are well-defined and implemented before AI deployment.
Q: How do you manage data risk in AI models?
- Rob Server suggested focusing on data classification and access control mechanisms. By understanding what data is used and who has access to it, organizations can better manage risks associated with AI models.
Summary
Generative AI stands out as a game-changer in data security, offering unparalleled capabilities in data discovery, risk management, and regulatory compliance. By leveraging this technology, organizations can safeguard sensitive data more effectively and ensure they are well-prepared to meet future security challenges.
At Spirion, our mission is to protect what matters most— your sensitive data. We are committed to providing cutting-edge solutions that help organizations navigate the complexities of data security with confidence and ease.
For more information, read the key insights from each of the C-Suite executives on this webinar’s panel.