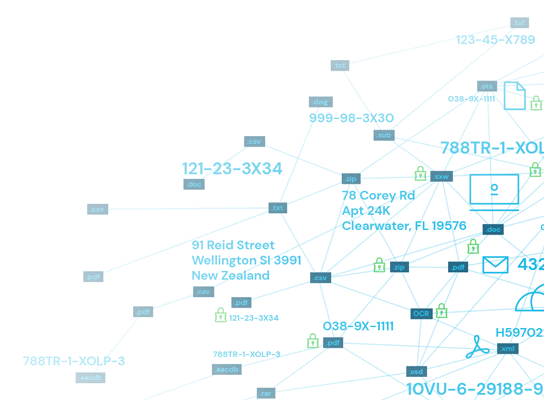
Data Lifecycle Management (DLM)
- What is Data Lifecycle Management?
- Data Lifecycle Management (DLM) vs. Information Lifecycle Management (ILM)
- Brief History of Data Lifecycle Management
- Main Purposes of DLM
- Challenges to Data Lifecycle Management
- Risks of Operating Without DLM
- Top Business Benefits of DLM
- Data Lifecycle Management Stages
- DLM Best Practices
- How to Enhance DLM with Data Privacy Compliance
Today’s organizations are increasingly data driven. They rely on data to run every aspect of their business — from acquiring customers to creating new products. Fueling the data-driven approach to business is one leading trend: the ever-expanding volumes of data from many sources, including customers, financial transactions, marketing campaigns, the cloud, mobile apps, emails, text messages, social media, Internet of Things (IoT) devices, and more.
To be useful, all data must be managed throughout its lifecycle — from creation to deletion. This can be challenging without the right data lifecycle management (DLM) program in place. Without a well-oiled DLM process, dealing with so much data can be frustrating and result in many missteps and lost business opportunities. To get the most out of their data and optimize the data deluge, and also keep it secure and in compliance, organizations need to understand DLM, the benefits, the challenges, and the best practices. This overview provides essential guidance for planning, deploying, and operating DLM programs.
What is Data Lifecycle Management?
Data lifecycle management has been defined in many ways — so much so it’s often misunderstood. It’s also often confused with other data management systems, especially information lifecycle management (ILM). Simply stated, DLM is the process, policies, and procedures of managing business data within an organization throughout its life — from creation through retirement. DLM is not a specific product, but a comprehensive approach to managing an organization’s data. It operates according to a policy-based system that manages the flow of information throughout its useful life across different applications, systems, databases, and storage media.
A well-run DLM process ensures that the organization understands, inventories, maps, and controls its data throughout as it’s created, modified, and stored. It will ensure that the most useful and most recent records are accessible with speed and ease. Much of this process can be automated into repeatable steps.
To understand DLM, think about it from the perspective of the movement of a single piece of data through the enterprise.
- A piece of data is captured through a sales process and entered into a database.
- The new data will either be accessed or shared for order processing, reporting, analytics, or some other use. Or it will sit in the database and eventually become obsolete.
- The data may have logic and validations applied to it during either process.
- At some point, it will come to the end of its useful life and be archived, purged, or both.
DLM is the process that pushes the data from one stage to the next.
Data Lifecycle Management (DLM) vs. Information Lifecycle Management (ILM)
Data management can take many forms. One of the most common forms, along with DLM, is information lifecycle management (ILM). In fact, DLM often gets confused with ILM to the point that the terms are often used interchangeably. While they are similar, they are different systems and processes. Both can be valuable and necessary in an organization to optimize data management, forming different critical aspects of an organization’s overall data protection strategy.
In a way, DLM and ILM are two sides of the same coin. Simply stated, DLM deals with entire files of data, while ILM is concerned with what’s in the file. DLM isn’t concerned with the individual pieces of data within a given record, just with the record itself. ILM seeks to ensure that every piece of data included in a record is accurate and up-to-date for the useful life of the record. Products that support DLM manage general attributes of data files (i.e., type, size, and age), whereas ILM handles specific pieces of data, such as customer numbers.
The distinction between DLM and ILM is becoming more important since the advent of the European Union’s General Data Protection Regulation (GDPR): Right to be Forgotten came into effect in May 2018. With this regulation, customers can request that a company erase their information, and provide proof that it has been. ILM is the process that can locate individual customer’s personal data across an enterprise. With purposeful data lifecycle management, DLM and ILM can be modernized to meet new privacy laws.
Brief History of Data Lifecycle Management
In the past, organizations had to be much more focused on the lifecycle of data due primarily to the capacity storage constraints on hard drives and other storage mediums. Today, storage space has become much more available and less expensive in the cloud, so the quantity of data an organization can manage is nearly limitless.
Today, DLM is very different from when the practice of managing data began in the 1980s. The precursor of modern DLM was designed to solve the issues of that time. For example, one of the leading challenges was the duplication of sensitive customer data. The 1980s brought the introduction of random access storage (RAM), and with that enterprise businesses transitioned from sequential card punch and tape approaches to databases.
Today, big data is changing how DLM procedures are crafted. Companies are collecting more and more data, so that they can realize the business benefits of big data analysis. However, these large stores of data have increased the need for DLM to effectively manage it all and keep it secure.
Main Purposes of DLM
The purpose of DLM is to make every stage of the data lifecycle more visible so that organizations can improve data processing efficiency and security and reduce costs. A DLM program is beneficial to any organization that creates, uses, and stores internal and consumer data. There are a number of reasons why an enterprise corporation should implement DLM processes. Here are three leading benefits:
Processing Improvement
With a good DLM strategy, data is easily accessible, clean, and usable. This means data-driven processes can be executed more efficiently, so the organization can achieve greater agility and control over data.
Data Protection
A good DLM strategy helps to ensure that customer data is safeguarded. Because DLM defines how data is processed, stored, and shared, it can establish practices that prevent loss. As a result, businesses can mitigate the risk of data deletion, losses, and breaches.
Compliance and Governance
Each industry has its own stipulations for data retention. Implementing a sound DLM strategy helps businesses ensure they are compliant with laws and regulations, both locally and internationally. For example, data management has become increasingly important as businesses face compliance regulations from the Sarbanes-Oxley Act, which regulates how organizations must deal with particular types of data. As a result, businesses can mitigate the risks of non-compliance citations and penalties.
Challenges to Data Lifecycle Management
One of the main challenges facing companies is managing all of the data they create. This has become a critical issue as organizations transform into increasingly digital, data-driven enterprises. They are inundated with data on three fronts:
Volume of Data Sources
Mobile apps and devices generate massive amounts of data from new sources, such as images, audio, and video. The new data provides enterprises with opportunities to gain additional insight and value. In the past, companies were constrained by how much data they could process and store. Now they have access to unlimited storage.
Ubiquity of Data Locations
Today data is everywhere — and companies need to capture it all, including customer data, financial transactions, purchase histories, customer journeys, marketing campaigns, service inquiries, market feeds, social media streams, software logs, and text messages. The more data a company can access, the more data analysis they can conduct to inform their decision making on everything from product development to marketing.
User Demand for Access
Employees within an organization are increasingly using data to fulfill their team’s missions, including decision support, business outcome forecasting, and creating new solutions. The more data they can access the smarter decisions they can make.
Risks of Operating Without DLM
But organizations also face enormous challenges in ensuring that they can protect, preserve, and manage data at every stage of its lifecycle. Without a coherent and comprehensive DLM approach, organizations face huge risks, including:
Spiraling Storage Costs
The unprecedented sprawl of data across organizations, including rapid expansion and growth of unstructured data, leads to higher storage costs.
Security Breach Risks
Data security is an ever-present risk in businesses of all sizes. Any breach opens a Pandora box of problems for an organization.
Noncompliance with Regulatory Requirements
A growing number of new and existing regulations are placing a higher penalty on noncompliance with data privacy regulations.
Inability to Respond to E-Discovery Demands
Expectations are high for organizations to immediately respond to electronic discovery requests. Any delay could present a risk to the organization.
Missed Opportunities
The potential for missed opportunities caused by an inability to leverage the strategic value of data through business intelligence and analytics is high. Most organizations work diligently to mitigate this risk.
Top Business Benefits of DLM
Managing data throughout its lifecycle has become a strategic imperative for many organizations. Faced with staggering data growth, leaders recognize the need to adopt solutions and practices that not only control costs and reduce risk, but also effectively manage a wide variety of data types to achieve competitive business advantages. Companies that deploy DLM as a strategic initiative and ensure high-quality handling of the information they generate gain several business advantages.
- Control Costs
A DLM strategy places value on data as it moves through the stages of its lifecycle. Once data is no longer useful for production environments, organizations can leverage a range of solutions to keep costs down, including backup, replication, and archiving. For example, it can be moved to less-costly storage on-premises, in the cloud, or in a hosted off-site tape vault. - Accelerate Time-to-Value
Organizations must be able to deliver data, services, and applications faster than ever before to satisfy customers’ and employees’ demands. A DLM strategy makes everyone more productive — from customer sales reps to service technicians. This not only gives teams the ability to find and access data whenever it’s needed, but also eliminates the information silos that prevent essential business collaboration. The extraction and maintenance of information throughout the data lifecycle ensures updated values are always available. - Data Security and Compliance
A data security or privacy breach can be devastating to an organization. It’s not only costly, but also can result in long-term damage to a brand’s reputation and customer goodwill. Security and privacy is much more complex in today’s business environment. A DLM strategy will incorporate data protection as one of its core capabilities, ensuring a good data protection infrastructure. - Data Usability
With a DLM strategy, IT can develop policies and procedures to ensure all data is consistently tagged and indexed so it can be found and accessed whenever it’s needed. Establishing enforceable governance policies future-proofs the value of data for as long as it needs to be retained. The availability of clean, useful, and precise data available to all users increases the agility and efficiency of company processes. - Improve Business ProcessesMapping data to business processes delivers a wide range of advantages, including:
-Identify and reduce process and data bottlenecks
-Control redundancy through more accurate identification of duplicate records
-Minimize or eliminate unwanted changes to data content
-Improve consistency, reliability, and access to needed data
-Improve the ability to perform root-cause analysis
-Trace data lineage across the customer demographic lifecycle
-Improve management of historical data
Data Lifecycle Management Stages
To understand DLM, it’s necessary to know the phases in the data lifecycle. While there is no industry standard for enterprise DLM, most experts agree that the data lifecycle includes these six stages: creation, storage, use, sharing, archiving, and destruction.
Stage 1: Data Creation
Data acquisition and capture occurs at the beginning of the cycle when an enterprise organization obtains new, vetted information, including creating data internally, purchasing third-party data, and collecting data as it streams from apps. Data is created in multiple formats, including Word documents, Excel spreadsheets, PDFs, emails, texts, images, and more.
Stage 2: Data Storage
Data storage in DLM refers to implementing data redundancy and security strategies on active data (versus inactive data that is archived), as well as storing data in such a way that it can’t be accidentally altered. Data storage must be compliant with contracts and regulatory laws. That may mean only storing it on servers or only storing backups on encrypted drives.
At this stage, the stored data is accessible only to assigned personnel. It’s common to specify the sensitivity of the data, including private, sensitive, restricted, or public. This protects the organization’s intellectual property as well as its customer relationships.
This stage also manages data recovery plans. If a failure occurs, organizations should have a plan for continuing to access the data, such as a temporary backup on a hierarchical storage management system.
Hierarchical Storage Management (HSM)
HSM is one type of DLM product. The hierarchy represents different types of storage media, such as RAID (redundant array of independent disks) systems, optical storage, or tape, each type represents a different level of cost and speed of retrieval when access is needed. Using an HSM product, an admin can establish guidelines for how often different kinds of files need to be copied to a backup storage device. Once the guideline has been set up, the HSM software manages everything automatically. Typically, HSM applications migrate data based on the length of time since it was last accessed, while DLM applications enable policies based on more complex criteria. Storage media for HSM includes:
Stage 3: Data Use
At this stage, data usage ensures the record meets certain validations to be accessible for users. When it comes to data use, DLM defines who can use the data and for what purposes.
Stage 4: Data Sharing
When data is published, it can be made available to people outside of an organization’s system, such as an invoice that uses customer data as a matter of record. Data is constantly being shared. Employees, users, customers, friends, executives, and board members either share data in the platforms they’re provided or by other methods. The more people who share data via unofficial methods, the higher the risk that it’s not compliant with governance and legal or policy regulations.
Stage 5: Data Archiving
In this stage, data undergoes an archival process that ensures redundancy. Active archives are an ideal storage method for organizations that tend to store large volumes of data and who need access to the information from time to time. Archived data isn’t active. So it can be stored on drives that aren’t on the network, but accessible for ad hoc reporting and analytics. DLM strategies define when, where, and for how long data can be archived. This will vary depending on what the data is. It may be something that has legal regulations, such as personal, research, or medical data. Or it may simply be internal company documents.
Stage 6: Data Destruction
In the final stage of the lifecycle, data is purged from the records and destroyed.
DLM Best Practices
One of the most important responsibilities for the IT team is to develop and execute an effective DLM strategy that uses best practices to protect, preserve, and manage all of the data across the enterprise at all times. Recommended best practices include the following:
Deploy Automated Solutions to Run DLM
DLM is essential to many vital business actions. To facilitate these actions and their underlying decision making, the best DLM strategies need to be repeatable, understandable, manageable, and automated. Achieving these goals is only possible when an organization deploys automated solutions that organize data into separate tiers according to specified policies, and migrate data migration from one tier to another based on those criteria.
Share the DLM Policy Across the Organization
Once the processes for handling data storage, management, backup, archiving, and deletion are defined, organizations need to share the process across the organization, so that everyone is aware of the new process, and stakeholders can implement the processes in a timely manner. Having clear-cut guidelines is the only way to get an entire organization onboard and participating in a new DLM program. Appointing a DLM champion can help field questions regarding the new policy and keep the organization on track with compliance and guide users.
Clearly Define Data Types
Organizations handle many different types of data. It’s vital to distinguish the types of data an organization manages. For example, customer data will need to be handled differently than accounting data. Identifying the type of data being stored and used is the starting point for outlining how to manage each type of data.
Implement a Robust Recovery Plan
Any file that lives on a physical storage device or computer is vulnerable to lose. To adequately protect data from loss and deletion, it’s essential to invest in a reliable backup system. With this solution, organizations will have the ability to retrieve any file at any time, thereby, eliminating permanent data loss.
Create a File-Naming Process
Losing data because it’s unsearchable is an easily preventable DLM failure. An organization should adopt a simple, yet thorough file-naming structure that allows anyone within the organization to find the data they need in seconds.
How to Enhance DLM with Data Privacy Compliance
Too often, much of the data created within organizations goes undiscovered — and, thus, unprotected. This creates a serious challenge for operating successful DLM programs. What’s more, it makes it difficult to ensure complete compliance with data privacy regulatory laws. What’s required to avoid these business problems is finding all data wherever it’s located across an enterprise (discovery) and tagging it with identifiers that make it easy to organize and find when needed (classification).
Here is how adding an automated data discovery and classification process works through the DLM process.
Step 1: Data Creation
Every minute of every day, organizations are creating ever-more massive stores of structured and unstructured data in multiple formats. A data discovery and classification program helps organizations keep it all organized, so that it’s both usable for business purposes and protected from privacy breaches.
Step 2: Storage Management
Once data is created, it needs to be protected with the necessary security controls. To deliver optimized data security, organizations need to enhance DLM with detailed data management reporting capabilities. Ideally, a data discovery and classification platform automated data security policies and workflows according to both internal policies and data privacy compliance regulations.
Step 3: Role-Based Security and Data Use
To ensure optimum data security, role-based security controls should be applied to all sensitive data via tagging based on internal security policies and compliance rules. Whenever a file is created, copied, edited, detached from an email, extracted from an archive, retrieved from cloud storage, or otherwise modified, it should be instantly searched, classified, and reported. A common data classification schema is to categorize data as public, internal, sensitive, or restricted.
Step 4: Data Sharing
Data is constantly being shared among employees, customers, and partners from different devices and platforms. As it’s shared, it moves between a variety of public and private storage locations, applications, and operating environments. Data should be discoverable no matter how many times it’s moved, copied, and shared — and where, including within Windows, Mac OS X, Linux, emails servers, MS Office 365, websites, and images. Employees should be notified about sensitive data in files, so that they can take appropriate privacy actions.
Step 5: Archive Solutions
Most data is eventually archived within an organization’s storage systems. Once archived, it’s important for the data to be both available and protected according to industry compliance rules. Organizations need to ensure that archived data is accurately classified according to all pertinent internal and external guidelines.
Step 6: Permanently Store Data
Significant amounts of data need to be destroyed to reduce the storage burden and improve overall data security. It’s essential for this process to be performed correctly to guarantee good data management. Data classification and local or international laws will mandate its disposal method. Before destroying any data, it is critical to confirm whether or not there are any policies in place that would require you to retain the data for a specific period of time.
Spirion’s proprietary AnyFind™ data-discovery technology ensures that all data is discovered and classified both on-premises and in clouds, including personally identifiable information (PII), protected health information (PHI), and payment card industry (PCI) data — as well as an organization’s confidential information and intellectual property. As data is created, it is instantly searched, classified, and reported, thereby, setting the stage for optimized security and compliance.