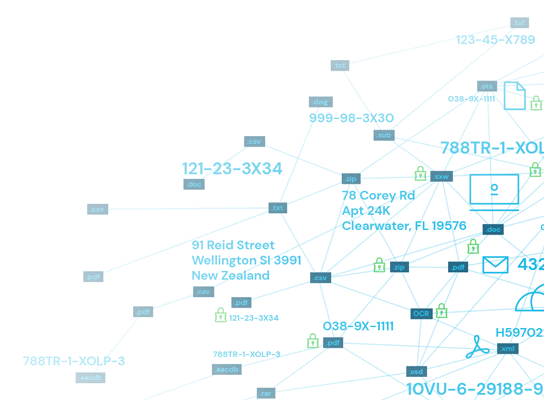
Data Loss Prevention Guide
In today’s business environment, organizations handle a vast quantity of data that is persistently at risk of loss and leakage, either intentionally (i.e., cyber criminal breaches) or accidently (i.e., employee errors). With the decreasing cost of data storage, more information is being stored than ever before. Much of this information is unorganized, untagged, and unused dark data, but even when an organization is aware of what information they have available, there are many vulnerabilities that exist. Modern storage can be accessed from remote locations, data may be spread across various cloud services, and physical devices like laptops and mobile phones may contain sensitive information. All of these factors and more exacerbate the risks of accidental or intentional data loss.
In this high-risk environment, it’s becoming increasingly difficult to ensure that all of this data is secure. However, organizations still need to enact effective security policies to protect data from loss and leakage. Data loss prevention (DLP) is one of the measures that organizations are deploying. DLP programs provide organizations with a set of processes and tools to detect data loss risks, understand and manage the risks, and prevent data losses and leakage. By deploying robust DLP programs, organizations can better manage data privacy and security while also improving regulatory compliance.
Understanding the value of DLP begins by understanding the issues driving DLP growth, including the causes of data leakage, recent changes to compliance regulations, the complex DLP solutions landscape, and more. With this knowledge, organizations will be better equipped to keep their sensitive data safe from all risks. This guide provides a comprehensive overview of DLP, including what it is, data loss challenges, and how to leverage DLP technologies to expand and enrich your organization’s data privacy and security programs.
Defining Data Loss Prevention
DLP processes are information protection practices created to protect sensitive or critical data in the corporate network from being erroneously or maliciously lost, misused, or accessed. DLP controls help mitigate the risk of data leakage, loss, and exfiltration by ensuring that sensitive information is identified and risk-appropriate controls are deployed to protect the information, while at the same time allowing organizations to access the data to conduct regular business. DLP is not a single piece of software, but an important component of a comprehensive data security and privacy program. To have an effective DLP strategy requires a comprehensive approach to data protection.
A brief history of DLP
DLP is not new. It launched into the world of cybersecurity in the mid-2000s. There was an initial spike in interest as companies sought solutions for the rapidly increasing risks of external hacking, the primary cause of data loss at the time. Early DLP solutions joined other technologies designed to protect networks from external threats and external data exfiltration, including firewalls, intrusion detection systems, vulnerability scanning, and penetration testing.
Early adoption of DLP technology was low, and results were often unsuccessful. DLP solutions were implemented only for limited areas, such as web and email monitoring, and not as integrated solutions. Additionally, early data loss prevention solutions could not capture the rising risks of internal data loss and leakage, a problem that began expanding at the same time. This required different solutions that focused on data handling within the organization in addition to data outflow. After several missteps, interest waned largely due to DLP technology’s complexity, cost, and inability to demonstrate business value.
By the late 2010s, organizations began taking a renewed interest in DLP for several reasons, including the challenges of securing an expanding universe of data and working within increasingly complex enterprises composed of cloud computing, mobile computing, and remote working. Further, new and stricter compliance regulations like the General Data Protection Regulation (GDPR) began demanding greater data privacy and security from organizations.
By this time, DLP technologies had matured into comprehensive solutions, providing more effective capabilities. These solutions have evolved to prevent, detect, and respond to the risks of sensitive data loss and leakage. As a result, DLP solutions are gaining traction as enterprises look for new ways to reduce the risk of data loss and leakage. In this environment, DLP has risen to become a top IT spending priority in many industries, and the data loss prevention market is expected to grow to $6 billion by 2026.
DLP categories
Organizations must protect these three categories of data:
Personally identifiable information (PII)
PII is any data that could potentially be used to identify a particular person. Examples include a full name, Social Security number, driver’s license number, bank account number, passport number, and email address.
Personal health information (PHI)
PHI is any piece of information in an individual’s medical record that was created, used, or disclosed during the course of diagnosis or treatment that can be used to personally identify them.
Intellectual property (IP)
IP is a category of property that includes intangible creations of the human intellect, including copyrights, patents, trademarks, and trade secrets.
Types of data loss and leakage
Data loss and leakage can be initiated by both internal and external sources, and either intentionally or accidentally. DLP protective measures need to address all areas to ensure that all data loss and leakage threats are prevented, including:
External data breaches
Today’s organizations are as at risk of massive data breaches by cybercriminals as ever before. In fact, as enterprises have developed more sophisticated tools to prevent attacks, cybercriminals have developed stronger ways to commit attacks. Many cyber attacks have sensitive data as their target. Attackers penetrate the security perimeter using techniques, like phishing, malware, or code injection, and gain access to sensitive data, which they then use to sell on the dark web or to commit even more cybercrime. As of 2022, the average cost of a data breach is $4.35 million.
Insider threats
Included in malicious attacks are breaches committed by insiders. Over the past decade, internal data losses from disgruntled or ill-intentioned employees have become a bigger risk. A malicious insider attempts to move data outside of the organization, often by abusing their permissions. Data leakage from insider sabotage, fraud, and theft is often referred to as data exfiltration.
System data leakage
Every day, large quantities of digital data flow outward from organizations’ networks and systems in the form of emails, data uploads, file transfers, instant messages, and more. This data can be lost or leaked by insecure information sharing processes, as is often the case when an organization fails to develop a comprehensive data security strategy.
Accidental human breaches
Unauthorized internal data leakage does not necessarily mean malicious. Many are accidental, such as unintentionally sending an email to the wrong recipient. This accounted for 24% of data breaches in 2019.
Main objectives of DLP solutions
Modern DLP solutions solve four main objectives that are common pain points for many organizations:
Protecting PII
DLP software solutions allow administrators to set business rules that classify confidential and sensitive information, so that it cannot be disclosed maliciously or accidentally by unauthorized end users. DLP solutions can also go beyond simple detection, providing alerts, enforcing encryption, and isolating data.
Staying compliant with regulations
DLP solutions can help organizations meet regulatory compliance rules by identifying, classifying, and tagging sensitive data, and monitoring activities and events surrounding the data. In addition, DLP reporting capabilities provide details required for compliance audits and Data Risk Assessments.
Protecting IP
Intellectual property theft can take many forms. From malicious insider attacks to theft from international governments, the loss of proprietary or confidential business information can cost millions of dollars. However, DLP solutions can mitigate some of these risks through a combination of security policies and tools.
Providing data visibility
A comprehensive enterprise DLP solution can help identify risks and track data across enterprises on endpoints, within networks, in the cloud, and on mobile devices. This provides visibility into how teams interact with the data, where it exists, who uses it, and for what purposes.
DLP and data lifecycle stages
Broadly speaking, DLP solutions target data at three levels of the data lifecycle:
1. Client Level (data-in-use)
Protects data as it is being used for day-to-day business operations, for example, modified, uploaded via a browser, or copied to the clipboard. DLP techniques include ensuring proper authentication and controlling data access rights granted to users, applications, and endpoint processes. Policies are defined and deployed, targeting endpoints used by employees for their day-to-day business operations. User activities that violate predefined policies are monitored or blocked by DLP agents installed in endpoint terminals.
2. Network Level (data-in-motion)
Helps ensure that data transmitted within the organization is not routed to improper recipients or insecure storage areas. DLP policies focus on data movements outside of an organization’s network. Data transmitted from one location to another is monitored and, if required, blocked by the system at network or email gateways. Transmitted data packets are inspected using deep packet-level review techniques to verify the nature of the content in-transit. Data transfers using email, web, and file transfer are verified against policies to prevent or detect sensitive data leakage.
3. Storage Level (data-at-rest)
Protects data that resides in databases, cloud repositories, computers, laptops, mobile devices, and other storage areas. Sensitive information stored in repositories is scanned based on specific rules, using crawlers to identify the locations and assess data sensitivity levels and location appropriateness according to policies. Data discovery and classification scans are used to tag the files and monitor their access. Often used in conjunction with more advanced data access governance and remediation tools. However, data-at-rest often represents one of the larger security gaps in many organizations, highlighting the need for proper encryption.
Data Loss Prevention Stages
Leading trends driving DLP industry growth
The expanding challenges to protect data are boosting the demand for DLP solutions. The data loss prevention market was valued at $1.21 billion in 2019 and is expected to reach $3.75 billion by 2025, at an annual growth rate of 23.59%, according to Mordor Intelligence. The leading trends driving this growth are larger cyberattacks, massive growth of data, more data storage units, security talent shortage, changing cybersecurity practices, and stricter compliance regulations.
More and larger cyberattacks
Data loss is a significant challenge within today’s enterprises, creating a cascade of risks from financial to reputational, and it’s getting worse every year. Cybercrime damages totalled nearly $7 trillion in 2021 with that number expected to grow to $10.5 trillion annually by 2025. Further statistics worth noting include:
- It takes an average of 287 days for security teams to identify and contain a data breach.
- Attacks against company networks increased by 50% from 2020 to 2021.
- Cybercriminals can gain access to an organization’s systems in more than 93% of cases.
- Ransomware attacks occur approximately every 11 seconds on average.
Massive expansion of data
The volume and variety of data is exploding across today’s business world. An IDC report predicts that businesses will generate 175 zettabytes of data by 2025 at an annual growth rate of 61%. This unprecedented sprawl means that data is stored in every nook and cranny of enterprises’ IT infrastructure.
As data volume and variety explode, resources become more ineffective because data and information assets are harder to find. No one knows which files contain PII, IP, or other valuable and regulated content. As a result, organizations struggle to protect information, comply with legal mandates, weed out duplicate and redundant data, and empower employees to find the content they need to do their jobs.
The scope and definition of sensitive data has grown over time, further necessitating clear data classification policies. Sensitive data now covers intangible assets, for example, business methodologies and pricing models.
More places to protect data
Data flows in and out of organizations to partners, customers, remote employees, and other legitimate users. Employees use multiple authorized and unauthorized communication channels to send data, including email, instant messaging, shared online folders, collaborative software, texting, and social media. Employees also store data in multiple places, including desktops, laptops, notebooks, smartphones, file servers, legacy databases, and the cloud. All of this activity leads to a lack of data visibility and, therefore, is high risk for loss and leakage.
Security talent shortage
Many businesses are finding it difficult to fill security-related job positions. In surveys by ESG and ISSA, 43% of organizations were affected by cybersecurity talent shortages. The shortage makes automated tools like DLP more attractive, because they can perform tasks automatically that staff previously did manually.
Changing cybersecurity roles
To manage the ever-more sophisticated world of data privacy and security, organizations are modifying their security infrastructures in a number of ways. Two notable changes include a new data privacy role and changes to an existing role.
- Data Privacy Officer — A Data Privacy Officer (aka, Data Protection Officer or DPO) is a relatively new position within cybersecurity that came into being with the advent of the European Union’s General Data Protection Regulation (GDPR), which requires organizations to have a person specifically in charge of data privacy.
- Chief Information Security Officer — Overall, organizations today have appointed more Chief Information Security Officers (CISOs) to manage cybersecurity programs. They report to CEOs, and CEOs want to know how they are preventing data leaks. DLP policies provide visibility and report on the status of organizational data protection.
Stricter compliance regulations
Countries around the world are enacting new and modified data protection laws that impose more stringent requirements on organizations handling sensitive data. These laws include significant penalties for noncompliance with the regulations. DLP strategies and tools help mitigate and remediate data loss as well as address specific requirements outlined by many regulations. (See more about compliance regulations below.)
DLP and regulatory compliance
Citizens across the world are demanding that governments take data privacy seriously, and legislators are responding at a more rapid pace. With more states and countries adopting comprehensive privacy regulations, data subjects have more control and transparency relating to their personal information collected by businesses. This rapidly expanding regulatory landscape means it’s more important than ever for organizations to pay attention to data protection and data privacy initiatives.
All compliance regulations share the same fundamental goal of ensuring privacy rights to protect an individual’s identity and personal information. The following four data categories represent the main categories of compliance regulations impacting today’s organizations.
Healthcare data regulations
Any organization dealing with individuals’ PHI must comply with one or more of the following regulations responsible for healthcare providers, health plans, and companies that collect health data from employees.
- Health Insurance Portability and Accountability Act (HIPAA) — The HIPAA is a federal law that requires the creation of national standards to protect sensitive patient health information from being disclosed without the patient’s consent or knowledge. Types of data include traditional PII, as well as diagnosis and health insurance information.
- Health Information Technology for Economic and Clinical Health Act (HITECH) — The HITECH Act was created to promote the adoption and meaningful use of health information technology, such as electronic health records (EHR) and supporting technologies in the U.S. to promote the adoption and meaningful use of health information technology. When a healthcare organization implements an EHR system, the act gives patients in those practices (or third parties they designate) the right to obtain their PHI in an electronic format (ePHI).
Payment card data regulations
Anyone taking credit card payments or handling credit card data for individuals faces requirements for data security, including access control, firewalls, encryption, and software and hardware security, plus other issues such as penetration testing, skimming, phishing, risk assessment, and data breach response. The following regulation is the leading credit card compliance rule.
- Payment Card Industry Data Security Standard (PCI DSS) — The PCI DSS is a set of security standards designed to ensure that all companies that accept, process, store, or transmit credit card information maintain a secure environment.
Geographic data regulations
New geographic regulations are upping the ante when it comes to keeping individuals’ personal data private and giving them new ways to access their data. The newest geographic regulations may offer individuals satisfaction that the personal data companies collect about them is secure and kept private. But companies can struggle to put the proper security protocols and procedures in place for two main reasons. First, these regulations present different requirements in different countries that need to be clearly understood, and second, these regulations focus on objectives, but don’t provide a clear way to achieve compliance. The two newest geographic regulations impacting data security programs in today’s organizations are GDPR and CPRA.
- General Data Protection Regulation (GDPR) — The GDPR entered the world to protect EU citizen’s data in May 2018. Since then, businesses have adjusted their security programs to encompass the regulation’s stricter data privacy rules. The GDPR exposes multinational organizations to hefty financial penalties, additional rules for disclosing data breaches, and increased scrutiny if they fall into non-compliance. Case in point, the EU fined Google a record €2.4 billion (about $2.7 billion USD) for antitrust violations.
- California Privacy Rights Act (CPRA) — The California Privacy Rights Act is a piece of data privacy legislation passed in November 2020 to go into effect July 1, 2023. This act builds on its predecessor, the California Consumers Privacy Act (CCPA), and requires businesses located anywhere who do business with California residents to follow strict guidelines related to security, privacy, and transparency in regards to consumer personal information.
- Other U.S. State Laws — States differ on their data privacy and IT security compliance laws. For example, Nevada, Minnesota, and Washington have their own laws that create liability in certain situations for businesses that handle credit card transactions and are not in compliance with PCI-DSS. Also, 46 U.S. states have their own data breach notification laws.
Cost-benefit of deploying DLP
To ensure they are obtaining a high value proposition for a DLP deployment, organizations can conduct a cost-benefit analysis, comparing the cost of the solution investment, data loss, and benefits to the cost of a data breach. The global average cost of a data breach is at an all-time high of $4.35 million in 2022. The increase was driven by the multi-year financial impact of breaches, increased regulatory actions, and the difficult process of resolving cyber attacks. The report takes into account hundreds of cost factors from legal, regulatory, and technical activities to loss of brand equity, customer turnover, and drain on employee productivity. Other findings on the high cost of data breaches included:
- Breach costs have increased approximately 13% in just the last two years.
- Approximately 60% of organizations have been driven to raise the costs of goods or services provided as a result of a breach.
- Nearly 80% of critical infrastructure have failed to implement Zero Trust Architecture, the only way to effectively meet modern security needs.
- Compromised credentials were the cause of 19% of breaches, while phishing was the cause of 16% of breaches. Phishing also caused the costliest breaches with an average of $4.91 million per incident.
These figures show the high cost of data breaches and the need for organizations to reduce their cybersecurity risks and improve their security posture. Data protection processes, like DLP, should be an integral part of every organization’s information security program to avoid the high costs of data breaches.
DLP Solutions
How DLP Technologies Work
The DLP software solution marketplace is segmented on several levels, including by deployment type (e.g., on-premise, cloud-based), solution type (e.g., network DLP, endpoint DLP, storage-based DLP), end-user industry (e.g., IT, telecom, finance, insurance, healthcare), and geography (e.g., local, state, country). To detect and prevent data breaches, exfiltration, and unwanted destruction of sensitive data, DLP technologies perform the following basic automated functions:
Classifies data
Identifies various types of data in the organization by the levels of protection they require, including regulated, confidential, and business-critical.
Monitors and controls
Monitors the flow of data as it is accessed and shared by end users to identify any policy violations in a wide variety of locations, filters data streams on corporate networks, and monitors data in the cloud to protect data-at-rest, in-motion, and in-use, and to provide visibility into data and system access of:
-Emails and texts
-Servers
-Endpoints
-Cloud storage
-Shared applications
-Mobile devices
-Websites
-Social media
-PrintersIdentifies violations of policies
Defined by organizations or within a predefined policy pack, systems identify data leaks that are anomalous or suspicious.
Enforces remediation
Takes pre-defined actions to prevent end users from accidentally or maliciously sharing data, such as:
-Alerting users and admins
-Quarantining suspicious files
-Encrypting data
-Blocking traffic outright
-Filtering data streams to restrict suspicious or unidentified activityCreates reports
Provides logging and reports for compliance, auditing, forensics, and incident response purposes that identify areas of weakness and anomalies.
Types of DLP Software
There are three main types of DLP solutions from which organizations can choose based on their needs:
Network DLP
This solution traces, monitors, analyzes, and reports on all of the data flowing through ports and protocols used on the network to detect sensitive data sent in violation of security policies. It secures all network communications, such as email and web applications, by controlling and protecting information flow over the network using techniques like automatic data encryption. Network DLP’s benefits include:
- Protects an organization’s network processes, such as web applications, emails, and FTP.
- Monitors data as it moves throughout the network.
- Maintains a database that provides details on which data is being used and who’s using it.
- Provides visibility into all data-in-transit on a network.
Endpoint DLP
This solution deploys agents installed on all workstations and devices used by employees to monitor and prevent the transfer of sensitive information, such as on endpoints and standalone storage devices. As a result, data can be protected independent of the network. For example, endpoint DLP can encrypt all data that is transferred to portable devices, or enable admins to scan for sensitive data-at-rest on computers company-wide and take remediation actions if data is being stored or handled improperly. Endpoint-based agents can control information transfer between users, groups of users, and external parties. Some endpoint-based systems can block attempted communications in real-time and provide user feedback. Endpoint DLP’s benefits include:
- Installs as an agent on endpoint equipment and prevents data leakage from the endpoints.
- Provides visibility into data stored on endpoints that are physically located inside and outside the organization.
- Monitors workstations, servers, and mobile devices, including laptops, mobile phones, external hard-drives, and USB disks.
Storage DLP
This solution provides control over information that employees retain and share, and sends alerts when information is at risk of being exposed to outsiders, such as data in the cloud. Storage DLP benefits include:
- Provides information about data stored and shared by the users in an organization’s network.
- Provides views of sensitive files shared and stored on the network.
- Provides visibility into stored data via on-premise storage equipment and cloud-based storage.
Popular DLP Tools
There are many DLP solutions available in the marketplace. These are some of the most popular options:
Symantec DLP
This scalable software suite gives organizations the ability to see how and where information is kept across their enterprise. It monitors mobile, cloud, and endpoints, and is especially effective when employees are offline.
McAfee DLP
This software solution monitors data on premises, in the cloud, or at endpoints, where it protects intellectual property and supports compliance by protecting all sensitive information.
Check Point DLP
This technology educates businesses and individuals so that they can act efficiently and quickly to prevent data loss. It includes a central management console, and easy implementation using preconfigured rules.
Digital Guardian DLP
This software, available as a cloud-based or on-premise system, is compatible with Mac, Windows, and Linux endpoints, and can manage a large number of workstations.
How to Develop a DLP Policy
Technology is just one component of a comprehensive DLP solution. Effective data security requires that organizations create DLP policies and establish best practices for handling and storing sensitive data and handling security violations. The following list provides tips for setting up an effective DLP policy.
Classify and interpret data
Identify which information needs to be protected by evaluating risk factors and how vulnerable the data is. Invest in tools that can accurately classify and interpret data, because this is the foundation for implementing a suitable data protection policy.
Track unmanaged devices
Keep track of the unmanaged devices that contain sensitive information. These devices might include endpoints, servers, removable storage, and cloud storage, all of which can act as departure points for sensitive information.
Allocate roles
Involve the right people with defined roles and responsibilities from the inception of a DLP solution program. Building out role-based DLP rights and duties will provide checks and balances. The DLP team should include representatives who are responsible for data protection, data owners, and people from key functions, IT, and various business units. Define each individual’s role and responsibilities in the organization’s DLP strategy.
Involve leadership
Effective management is key to ensuring a DLP program’s success. To be successful, policies must be enforced at the organizational level.
Educate stakeholders
Ensure all stakeholders and data users are aware of the DLP program, and what they need to do to safeguard organizational data. Policies and procedures should provide clear guidance to employees on appropriate and inappropriate data loss prevention practices.
Document the DLP strategy
This will provide clarity, both at the individual and organizational levels, as to what is required and how the policy is to be enforced.
Secure the most sensitive data first
Start by identifying the data that creates the biggest risk to the business. The initial pilot implementation should be restricted to a region or division. Then a phased approach, prioritizing the modules and targeting key end points, provides an opportunity to learn from experience before wider deployment. An implementation road map should be planned, with appropriate milestones and checkpoints to review progress.
Automate as much as possible
Manual DLP processes are inherently limited in their scope and the amount of data they can cover. Automated systems provide limitless capabilities and capacity.
Establish success metrics
Determine the key performance indicators (KPI) that should be measured, and monitor them closely to determine the DLP program’s success, including percentage of false positives, number of incidents, and Mean Time to Response. Share these metrics with your organization’s leadership to show the positive impact of DLP and reinforce its business value.
Choose complimentary security solutions
One technology alone isn’t enough for today’s complex threat landscape. Organizations must protect networks, endpoints, clouds, and users, and the best way to achieve this goal is through the deployment of a multi-layered security approach that includes several integrated solutions.
DLP Integration Options
The technological means employed for dealing with data leakage incidents can be divided into four general categories: standard security measures, designated DLP systems, intelligent security measures, and access control and encryption. Ideally, organizations take a layered approach to DLP. This includes integrating additional data security applications.
DLP solutions are great at monitoring data flows and securing data from known threat patterns. However, there are several critical data security functions that they cannot execute. The following applications are supplemental solutions that companies can integrate into their DLP programs to build out a comprehensive data privacy and security program.
Common DLP integration options include the following:
- Intrusion Detection System (IDS) — Guards computers against outsider and insider attacks by sending alerts about attempts to access sensitive data.
- Security Information and Event Management (SIEM) — Detects events that might constitute a data leak.
- Content Monitoring and Filtering (CMF) — Filters malicious content and websites that normal anti-virus and anti-spyware programs may miss.
- Information Protection and Control (IPC) — A solution that addresses the digital management of rights to access information, control over that data, and the integrity of the data.
- Extrusion prevention system (EPS) — The practice of stopping data leaks by filtering outbound network traffic and preventing unauthorized packets from moving outside the network.
- User and Event Behavioral Analytics (UEBA) — Identifies malicious insiders and attackers who act in ways that do not match any known patterns, or cannot be captured by DLP security rules.
- File security solutions — Safeguards data-at-rest and in-use, and detect leaks of file-based data.
- Antivirus software — Prevents attackers from compromising sensitive systems.
- Firewalls — Blocks access from any unauthorized party to systems storing sensitive data, and prevents the access of outsiders to the internal network.
- Data discovery — See below.
- Data classification — See below.
Why choose Spirion data loss prevention software?
Before organizations can launch DLP tools, they need to execute data discovery and classification. A data loss prevention solution cannot succeed if organizations simply try to prevent adversaries from accessing or exfiltrating data — they must first accurately find and classify their sensitive data. Data discovery locates all of an organization’s data across an enterprise. Data classification identifies the data and labels it according to predetermined categories — from not sensitive to highly sensitive.
That’s where Spirion comes in. To protect organizations from widespread data loss or leakage, the Spirion Governance Suite finds structured and unstructured sensitive data of all kinds, including PII and IP, everywhere it exists. This step is a vital part of an integrated DLP solution.
Spirion’s software integrates with most leading DLP programs and adds these essential data loss prevention capabilities:
- Identifies sensitive data — Searches for all sensitive and confidential information across an entire infrastructure, including images, databases, hosted and on-premise email applications, cloud storage, and network devices.
- Performs data classification — Delivers classification automatically with a highly accurate data identification engine.
- Continuously monitors data — Data is continuously being received and created, necessitating a DLP strategy that continuously monitors the enterprise for new instances of sensitive data in real-time.
- Remediates data — Data remediation allows organizations to reduce their sensitive data footprint by performing data encryption, shredding unneeded information, redacting toxic data, or quarantining files to more secure locations.
- Provides an intuitive central console — An easy-to-use graphical user interface lets users search, review and protect their sensitive data. The centralized console is home to reports, role-based access control management, real-time data identification, and data classification results.
Data loss is a pervasive problem in organizations around the world, including both inside and outside threats. Deploying a powerful DLP program helps ensure that sensitive data is protected. Well-developed DLP strategies are integrated with other leading data protection solutions and can help prevent accidental or intentional data loss incidents or data breaches as well as the business-draining results they pose.
If you have questions about DLP strategies or software costs, Spirion’s security experts are here to provide guidance on how to move forward with an optimal DLP deployment.