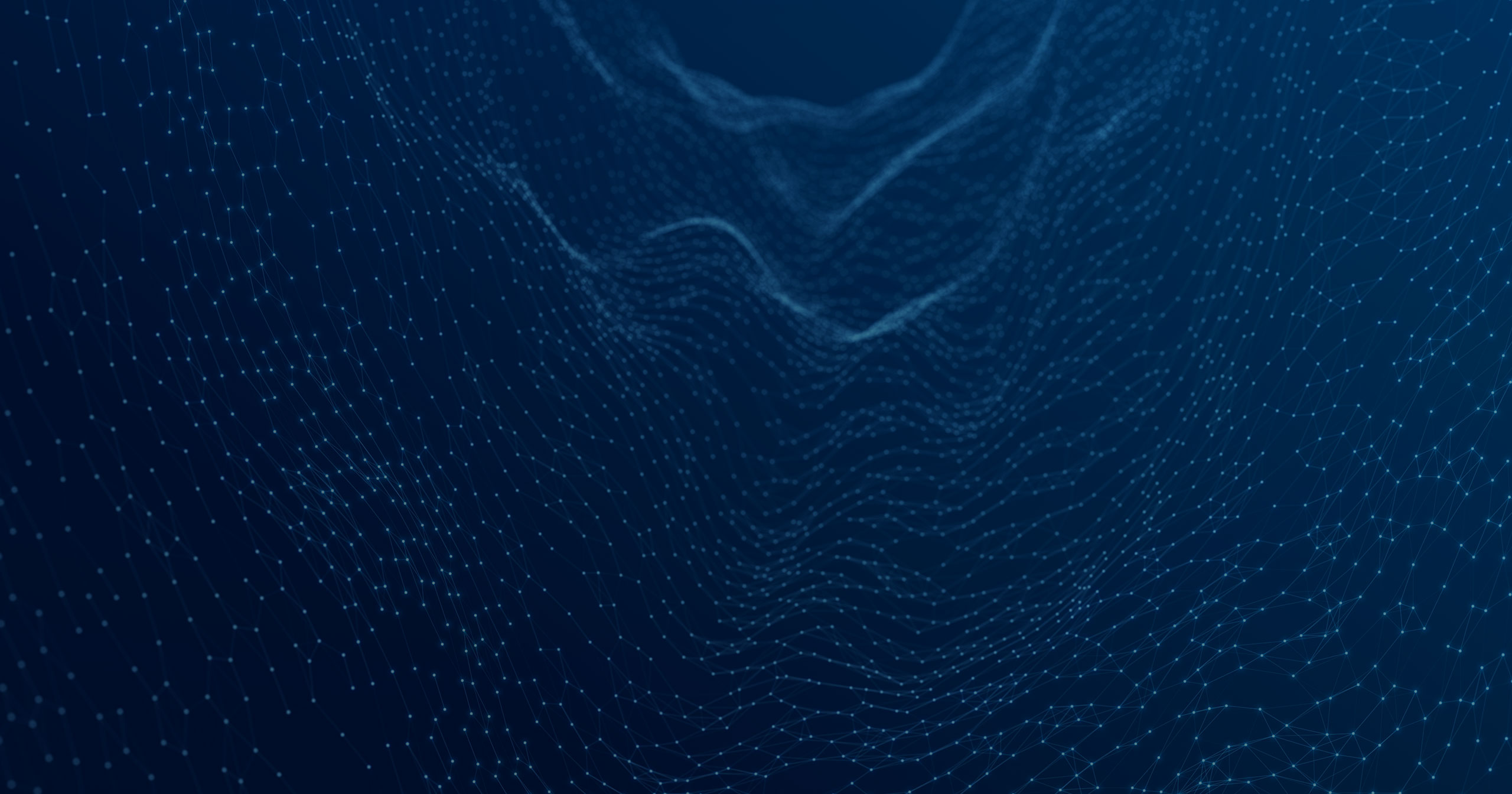
BY SPIRION
March 14, 2023
The year 2023 is shaping up to be a pivotal year for data privacy legislation. Five new “rights-based” privacy laws are going into effect, while many more states are considering similar policies.
While the coming months will present significant challenges for businesses of all sizes, trends indicate that more regulations should be expected in the future. This underscores the importance of addressing privacy law compliance obstacles expeditiously to avoid future financial and legal burdens.
In this, the second installment in our three-part series on data privacy and security predictions, we’ve gathered industry predictions and expert advice from our recent report and highlighted the most important privacy law trends you need to be aware of. You can read part one of the series here.
Data privacy legislation will continue be pushed forward at the state level
“More and more states are refusing to wait for the federal government to catch up to the privacy needs of consumers and businesses.”
– Todd Feinman, Founder, Board of Directors at Spirion | Data Privacy & Security Report
Though the American Data Privacy & Protection Act aimed to regulate consumer data on a national level, the law failed to pass despite bipartisan support. This has only increased fragmentation in an already confusing regulatory landscape.
The lack of a federal privacy law has led many states to take the enforcement of data privacy into their own hands. Though many of the new state laws surrounding privacy and data security address the same subject matter, inconsistencies in terminology, compliance requirements, and use restrictions will present significant challenges for businesses. Additionally, an anticipated federal law could only add another layer of complexity to an already convoluted environment.
Since compliance requirements may differ significantly among states and nations, businesses will need to adapt to the strictest policies on the books. This will include guidelines surrounding data processes like subject access requests (DSARs), a written communication from a data subject to an organization that asks which of the subject’s personal data is being stored, why and with whom it is being shared. Consumers are entitled to DSARs, thanks to privacy legislation like the GDPR and CPRA, and organizations are obliged to respond in a timely manner. In order to fulfill DSARs and uphold compliance, organizations need automation tools capable of accurately locating a subject’s data and supporting efficient workflows for receiving, processing, and completing requests without human error.
While classification is an essential part of your data security strategy, it has the potential to work against you if not executed properly. This is often the case with manual classification. With other automated platforms in your supply chain quickly churning out high volumes of data, it’s impossible to expect manual classification to keep up. Even if flawlessly executed, manual classification could be guaranteed, it’s extremely time-consuming and will inhibit your organization’s operational productivity.
Automated classification tackles these efficiency issues while also enhancing the efficacy of your greater security strategy through standardized tags/labels. From your data loss prevention (DLP) policy and authorizing user access to your Zero Trust approach and threat response procedure, having sensitive data labeled with common nomenclature ensures that all your sensitive data is properly defined in policies and procedures and can be processed accordingly by the tools used to execute them. This eliminates the security risks associated with inconsistent labeling at the user level and fortifies efforts to uphold compliance while data is both at rest and in motion.
“There are now more IoT devices within organizations than computers, and companies may not sufficiently understand their increased data privacy risks.”
– Debbie Reynolds, “the Data Diva,” Debbie Reynolds Consulting, LLC | Data Privacy & Security Report
Decentralized computing and an increasing number of endpoints will continue to represent security risks. In addition to cyberattacks, there are significant risks for noncompliance in many industries, including financial services (GLBA and PCI-DSS), healthcare (HIPAA), and education (FERPA). IoT devices represent a significant threat surface, and the need for proactive and automated data protection has never been more urgent.
In a similar vein, new technologies like chatbots AI software will also present a potential privacy risk for businesses. In an effort to keep up with competition, companies may feel pressure to implement technologies that they may not fully understand, which can further enhance risk. Given the scope of privacy laws on the books, it is imperative that organizations understand how information is being gathered, used, and shared with third parties. Unauthorized tracking, use, or sharing of data with third parties could have significant negative ramifications.
Privacy Enhancing Technologies (PETs) will enhance business operations
“The top privacy challenge of 2023: how to unlock value from data without increasing your risk or compromising your interests? In the year ahead, data-driven organizations will increasingly turn to Privacy Enhancing Technologies (PETs) to meet these needs.”
– Dr. Ellison Anne Williams, Founder and CEO of Enveil | Data Privacy & Security Report
Organizations have access to more data than ever before. While information represents incredible opportunity, this data must be leveraged securely and privately. Privacy Enhancing Technologies (PETs) will give organizations the tools they need to access this data securely and in ways not possible before.
A proper PET solution can help CISOs solve modern data security, privacy, and governance challenges with streamlined solutions. Where previous technologies required cumbersome extraction, translation, modeling, and mapping techniques, new products like the Spirion Sensitive Data Platform can go beyond traditional data classification limits to unlock insights in both content and business context to automate data classification policies.
From a compliance perspective, these automated processes offer greater control over enterprise data. With a more robust, responsive, and scalable classification solution, companies can:
- Classify each piece of information with purpose. This includes dynamic classification labeling, which updates classification labels when labels are modified or added.
- Categorize and tag data automatically based on sensitivity and existing information security policy and processes, regardless of how many times data is moved or copied.
- Integrate at-a-glance, user friendly icons and classification markers for each bit of data.
- Automatically label data based on its purpose for collection, the process through which it was collected, and its privacy level. From there, labels can be federated across the entire IT environment.
To learn more about contextual data classification, read our white paper: Context is everything.
Conflicting laws and regulations place additional burdens on businesses
“The increasing number of individual state privacy laws and the accompanying regulatory “creep” associated with each one amounts to incredible compliance burdens that will be difficult and costly for businesses to meet.”
– Thomas Besore, Privacy Compliance Consultant | Data Privacy & Security Report
Conflicting state privacy laws and regulations will place significant additional burdens on businesses. Potential challenges include:
- Compliance Costs. Businesses will need to devote significant resources to understand and comply with multiple and potentially conflicting state privacy laws and regulations, which will be costly and time-consuming.
- Legal Risks. Failure to comply with conflicting state privacy laws and regulations may expose businesses to significant legal risks, including fines and penalties, litigation, and reputational harm.
- Stifled Innovation. As an added safety measure, many businesses may choose to adopt more conservative approaches to data processing and use. While this can reduce regulatory risk, data may not be utilized to its full potential.
Businesses must remain agile and be able to quickly adapt to the new regulations to remain compliant, while also delivering the best customer experience. One way to do this is to streamline and simplify data classification activities across every type of data in every location across the IT environment. Automating this process from a single platform can help businesses quickly, reliably, and continuously update data classification to dramatically enhance their data compliance initiatives while also protecting their bottom line.
Automated compliance tools will increasingly fuel the way forward
Effective data classification can be elusive. Every piece of data within your organization represents a unique combination of business value and level of risk. As privacy concerns, cybersecurity threats, and compliance mandates gain intensity, the need for effective data classification is more urgent than ever. Classification systems help you set boundaries around data access, use, and modification, acting as a natural next step to protect data once discovery efforts are complete.
However, this approach includes its own challenges, such as:
- Systems that are too cumbersome to gain widespread adoption
- Tools that are not sophisticated enough to consider critical context, resulting in misclassification and potential business disruption
- Classification decisions that are based on internal politics and departmental preferences rather than evidence
- Inconsistent or non-comprehensive application of systems resulting in missed file types or locations.
Modern data lifecycle management requires modern solutions, and both IT and business leaders are increasingly seeking robust, automated data classification solutions to help keep their data safe and their businesses out of legal trouble.
Fortunately, new data classification solutions can automatically (and persistently) classify data, updating its tags as it moves through the data lifecycle. Now, any time data changes in any manner, for any reason, it can be classified with the appropriate tags for access, use, and archiving. Ultimately, an automated classification tool simplifies an increasingly complex, but vital, data security operation by:
- Unburdening IT teams of time-consuming manual work
- Significantly enhancing a company’s ability to comply with ever-changing compliance requirements
- Improving risk-related decision-making and responses with real-time data
In the event of a data breach, having compliant security measures in place based on accurate classification can even help to reduce the fines imposed by regulatory agencies.
Learn how contextual data classification can bolster compliance in our ebook: Future-proofing sensitive data privacy and compliance.
Prepare for an uncertain regulatory environment with help from Spirion
Trying to comply with constantly changing laws and regulations can feel like trying to hit a moving target. Instead, simplify your approach to compliance with Spirion’s Governance Suite.
The Governance Suite combines all of Spirion’s data security and privacy products into one powerful platform while retaining the customizability your organization needs to retain flexibility.
Accurately discover data wherever it lives, harness the power of automated classification, and efficiently comply with stringent regulatory policies like Data Subject Access Requests (DSARs). With a proactive approach to data privacy, your organization will be able to meet the demands of even the most complex regulatory guidelines.
See the solution in action to better understand the power of the Governance Suite. You can also contact us to speak with a member of our team and have your questions answered by a data security expert.